Table Of Content
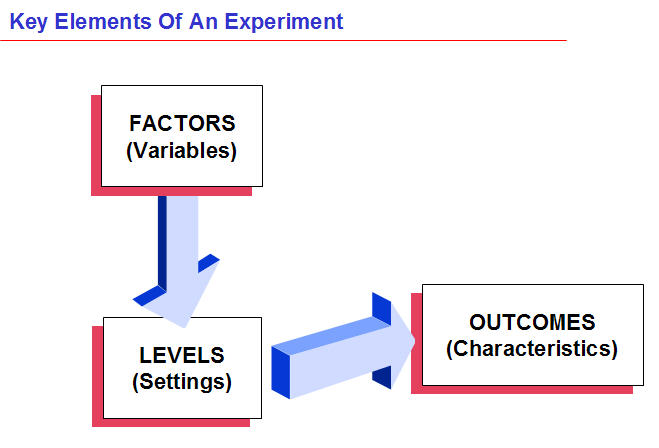
DOE also identifies and explores the interactions between those factors. This allows us to optimize the performance and robustness of our processes or assays. This is an essential component of any experiment that is going to have validity. If you are doing a comparative experiment where you have two treatments, a treatment and a control, for instance, you need to include in your experimental process the assignment of those treatments by some random process.
Products and services
Design of Experiments (DOE) offers a daunting compilation of types of design. As a beginner, understanding which one is right for your needs can feel like an impossible task. In 1950, Gertrude Mary Cox and William Gemmell Cochran published the book Experimental Designs, which became the major reference work on the design of experiments for statisticians for years afterwards. Around 1990 Six Sigma, a new way of representing CQI, became popular. Now it is a company and they employ a technique which has been adopted by many of the large manufacturing companies. This is a technique that uses statistics to make decisions based on quality and feedback loops.
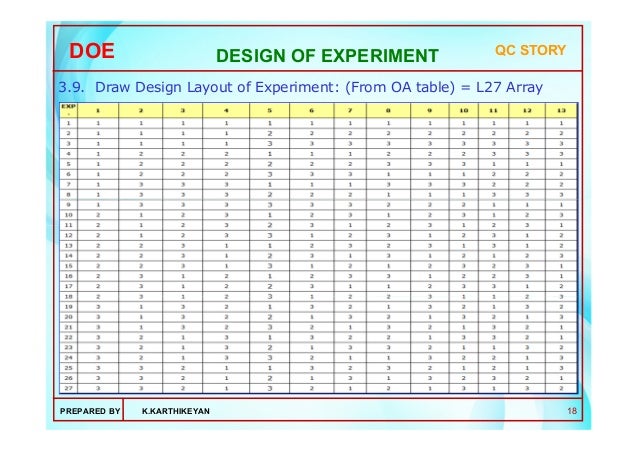
Book traversal links for 1.1 - A Quick History of the Design of Experiments (DOE)
Emergence is one reason biologists often lack well-developed, robust theoretical frameworks to guide their experiments. You can visualize, explore your model and find the most desirable settings for your factors using the JMP Prediction Profiler. Notice that none of them has trials conducted at a low temperature and time AND near optimum conditions. Experiments are likely to be carried out via trial and error or one-factor-at-a-time (OFAT) method. Plus, we will we have support for different types of regression models. For example, we can estimate what we call a linear model, or an interaction model, or a quadratic model.
Replication
Confounding is something we typically want to avoid but when we are building complex experiments we sometimes can use confounding to our advantage. We will confound things we are not interested in order to have more efficient experiments for the things we are interested in. Aliasing is particularly useful during screening to explore more factors without incurring a huge experimental cost.
Surprisingly the service industry has begun using design of experiments as well. Adjustments made based on the DoE findings significantly improved the consistency and strength of the welded joints. This optimization reduced material waste by 15% and increased production efficiency by reducing the instances of rework and testing required for quality assurance.
As you can already tell, OFAT is a more structured approach compared to trial and error. Run the second experiment by varying time, to find the optimal value of time (between 4 and 24 hours). Change the value of the one factor, then measure the response, repeat the process with another factor.
Taguchi Design of Experiments
Copyright © 2024 Elsevier B.V., its licensors, and contributors. All rights are reserved, including those for text and data mining, AI training, and similar technologies. For all open access content, the Creative Commons licensing terms apply.
Formulation and optimization of phytosomes of ethanolic extract of Viola tricolor flowers using design of experiment ... - ScienceDirect.com
Formulation and optimization of phytosomes of ethanolic extract of Viola tricolor flowers using design of experiment ....
Posted: Thu, 18 Jan 2024 16:19:28 GMT [source]
Applied Statistics: Data Analysis
Coaxial electrospinning of polycaprolactone – A design of experiments approach - ScienceDirect.com
Coaxial electrospinning of polycaprolactone – A design of experiments approach.
Posted: Thu, 29 Feb 2024 01:30:32 GMT [source]
We would have missed out acquiring the optimal temperature and time settings based on our previous OFAT experiments. DOE applies to many different investigation objectives, but can be especially important early on in a screening investigation to help you determine what the most important factors are. Then, it may help you optimize and better understand how the most important factors that you can regulate influence the responses or critical quality attributes.
You probably follow a recipe so there are many additional factors that control the ingredients - i.e., a mixture. What parts of the recipe did they vary to make the recipe a success? Probably many factors, temperature and moisture, various ratios of ingredients, and presence or absence of many additives. Now, should one keep all the factors involved in the experiment at a constant level and just vary one to see what would happen? This is one of the concepts that we will address in this course.
They are done by scientists and engineers, among others, in order to understand which inputs have a major impact on output and what input levels should be targeted to reach a desired outcome (output). Simply put, DoE is a way to collect information during the experiment and then determine what factors or which processes could lead to the desired result. Instead of concepts like power and aliasing, which are relevant to determining which effects are real, RSM uses concepts relating to the errors when making predictions in the relevant design space. Fractional factorial designs assume that while there may be many effects, only a few are important.
Taguchi, a Japanese engineer, discovered and published a lot of the techniques that were later brought to the West, using an independent development of what he referred to as orthogonal arrays. In the West, these were referred to as fractional factorial designs. These are both very similar and we will discuss both of these in this course. He came up with the concept of robust parameter design and process robustness. The engineering team applied a factorial design approach to investigate several factors simultaneously, including welding temperature, pressure, and duration. The objective was to identify the optimal conditions that consistently produced welds meeting strength standards while minimizing resource consumption.
It incorporates a lot of previous statistical and management techniques. Learn about quantitative and qualitative variables and explore different graph... The successful application of DoE in this context solved a critical manufacturing challenge. It demonstrated the method’s potential to make processes more efficient and sustainable.
By simultaneously testing multiple inputs, your DOE can identify significant interactions you might miss if you were only testing one factor at a time. With three variables, machine speed, fill speed, and carbonation level, how many different unique combinations would you have to test to explore all the possibilities? Which combination of machine speed, fill speed, and carbonation level will give you the most consistent fill?
By picking the right design in the beginning, you’ll be saving yourself tons of work and grief when it comes to the analysis. Which means that you don’t have to design a single experiment—or pick a single DOE design—that will answer all your questions. Stay with us and we’ll explain how the DOE stage is crucial for narrowing down which design you choose, and we’ll go through these basic categories in detail.
No comments:
Post a Comment